A Diffusion Model with A FFT for Image Inpainting
DOI:
https://doi.org/10.61702/MTPG8588Keywords:
Convolutional Neural Network, Diffusion model, Fast Fourier Transform, Image InpaintingAbstract
Diffusion models for image inpainting have been the subject of growing research interest in recent years. However, generating content that is consistent with the original images, especially for complex images with intricate details and structural information, remains a significant challenge. In this paper, we propose a diffusion model with an FFT (FFT-DM) to generate content that matches missing region texture and semantics to inpaint damaged images. Specifically, FFT-DM contains two components: a Denoising Diffusion Probabilistic Model (DDPM) and a Convolutional Neural Network (CNN). The DDPM is used to extract global features and generate image prior while the CNN captures more fine-grained details and predicts the parameters in the reverse process of the diffusion model. Notably, we integrate a Fast Fourier Transform (FFT) into the diffusion model to enhance the perception ability and improve the efficiency of the model. Extensive experiments demonstrate that FFT-DM outperforms current state-of-the-art inpainting approaches in terms of qualitative and quantitative analysis.
Downloads
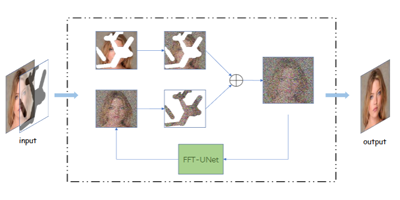
Downloads
Published
Issue
Section
License
CC Attribution 4.0